The general purpose of a dynamic factor model (DFM) is to summarize a large number of time series into a few common factors. In this paper Alex van de Minne, Marc Francke and David Geltner explore several DFMs on 80 granular, non-overlapping commercial property price indexes in the US, quarterly from 2001Q1 to 2017Q2.
They examine the nature and the structure of the factors and the index forecasts that can be produced from the DFMs. The paper considers specifications of one to four common factors. As a major motivation for the use of DFMs is their ability to improve out-of-sample forecasting of systems of numerous related series, they apply the DFM estimated factors in an Autoregressive Distributed Lag (ARDL) model to forecast individual market index returns. The authors compare for four markets the forecasts to those from a benchmark univariate autoregression. The results show that the DFM & ARDL model predicts the crisis and subsequent recovery really well, whereas the benchmark model typically extrapolates the past price trend.
Questions?
Do you have questions about this article or an idea for another interesting analysis? Please contact Marc Francke using the details below.Contact
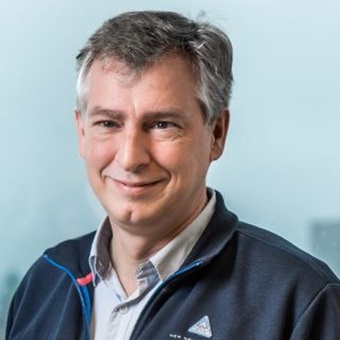